Small Language Models (SLMs) have been garnering significant attention in the tech industry, signaling a notable shift in focus.
This increasing interest stems from the growing recognition of the unique advantages that SLMs offer, particularly their efficiency, accessibility, and adaptability.
These characteristics are proving to be game-changers in a wide range of applications, making SLMs an attractive option for both large corporations and smaller startups.
What are Small Language Models?
The tech world, traditionally dominated by Large Language Models (LLMs), is now witnessing a transformative change. SLMs, with their smaller size and reduced computational requirements, are emerging as a powerful alternative. Unlike LLMs, which often demand extensive resources and infrastructure, SLMs can operate efficiently on standard hardware.
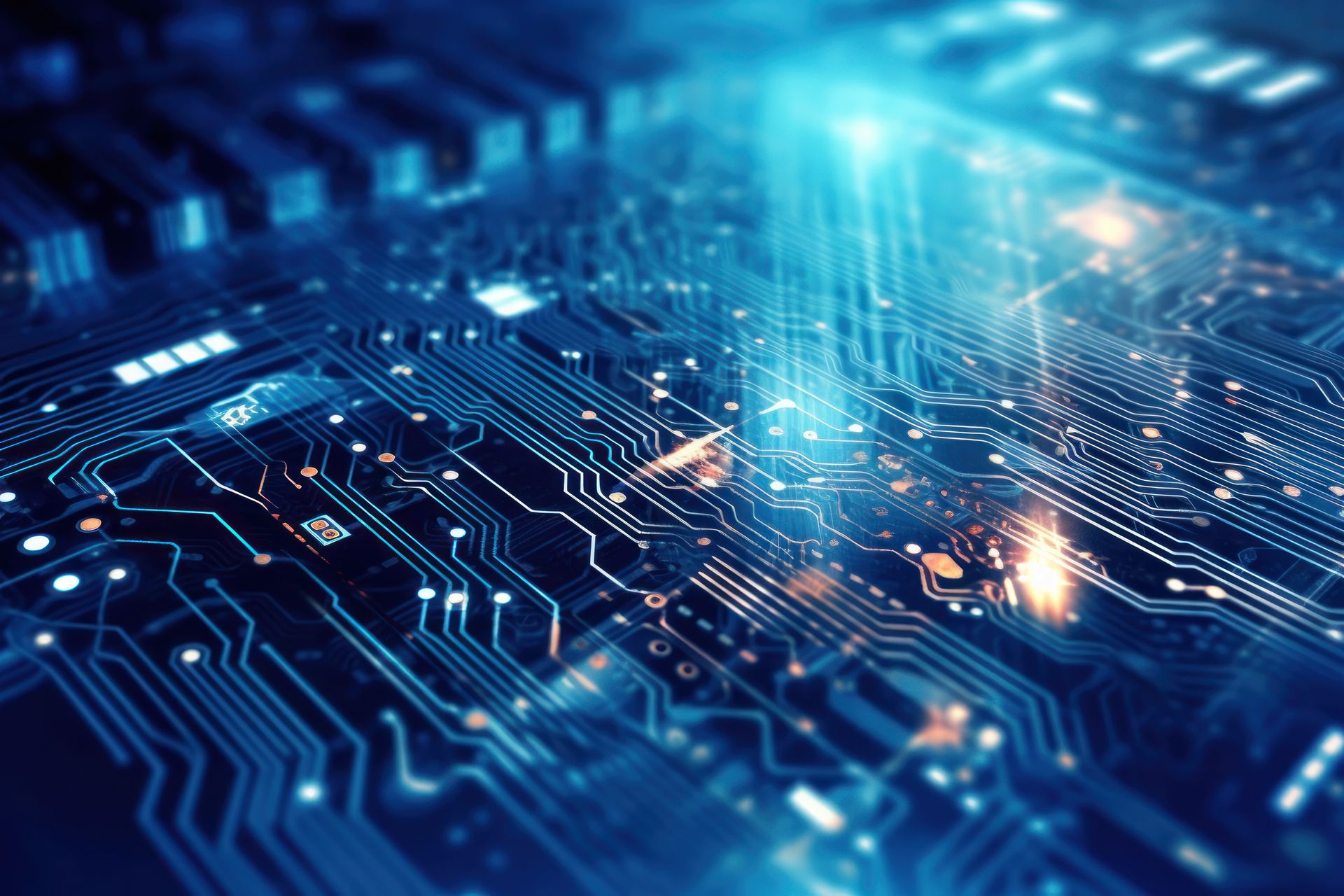
This shift towards efficiency is not just about cost reduction; it’s about democratizing access to cutting-edge AI technology. By requiring less computational power and memory, Small Language Models are making it possible for a wider range of organizations and individuals to leverage the capabilities of language models.
Customization and accessibility
The adaptability of Small Language Models is another key factor driving their adoption. These models can be easily fine-tuned and customized for specific tasks and domains, offering a level of flexibility that LLMs often lack. This adaptability is particularly valuable in scenarios where quick turnaround times and specialized solutions are required. Moreover, the accessibility of SLMs is opening up new possibilities for innovation.
With lower barriers to entry, developers and researchers are exploring creative applications of SLMs, from chatbots and virtual assistants to content generation and language translation.
SLMs in real-world applications
Small Language Models are already making their mark in various industries. In healthcare, they are being used to analyze medical records, assist with diagnoses, and even generate personalized treatment plans. In the financial sector, SLMs are powering customer service chatbots, fraud detection systems, and risk assessment tools.
The education sector is also benefiting from SLMs, with applications ranging from automated essay grading to personalized tutoring. The potential of SLMs seems limitless, and as research and development continue, we can expect to see even more innovative and impactful use cases emerge.
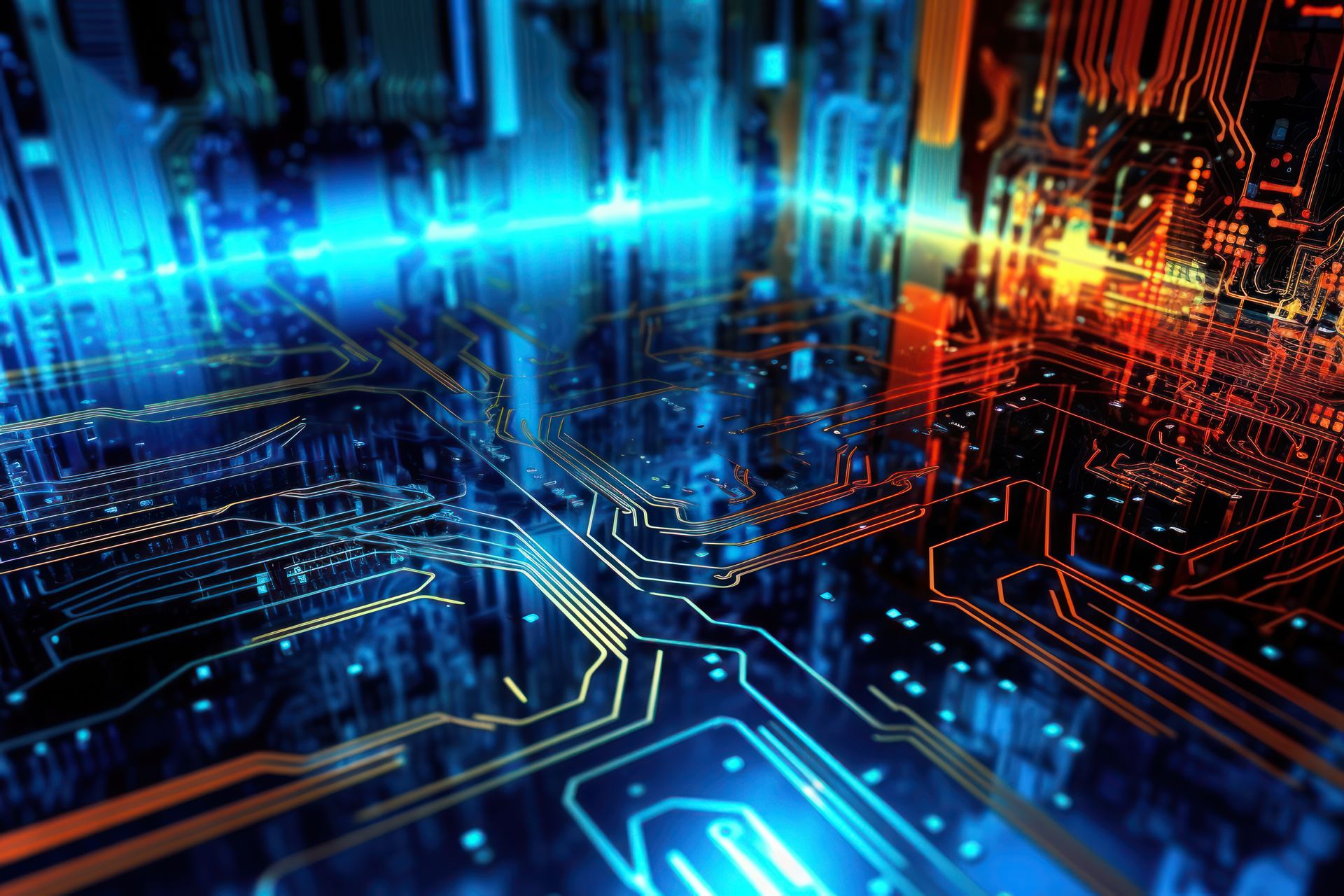
The path ahead
The shift towards SLMs represents a significant change in the tech landscape. It is not merely a trend but a fundamental change in how we approach and utilize language models. This change is driven by the need for efficiency, accessibility, and adaptability, which Small Language Models deliver in spades.
However, the journey is not without its challenges. As with any emerging technology, there are technical hurdles to overcome and ethical considerations to address. Ensuring fairness, transparency, and accountability in Small Language Models’ development and deployment will be crucial as we move forward.
Despite the challenges, the future of Small Language Models looks bright. The ongoing research and development in this area are rapidly advancing the capabilities of these models. We can anticipate SLMs becoming even more efficient, accurate, and versatile in the years to come. Additionally, the integration of SLMs with other technologies, such as edge computing and the Internet of Things (IoT), holds immense potential for creating intelligent, responsive, and personalized experiences.
Featured image credit: bnezoix/Freepik