A new study shows hard rock and hip-hop fans get less relevant song recommendations from Spotify algorithms.
The most used applications to listen to music, such as Spotify, Last.fm or Youtube, have algorithms capable of predicting and showing you new music that you might like. Simply put, it is a recommendation system through collaborative filtering: the apps record the artists and genres a user listens to and match these results with like-minded listeners to find out what others like.
Hard rock and hip-hop fans get less relevant song recommendations
But these algorithms are not perfect with something as subjective and human as artistic creation and musical tastes. For this reason, a team of researchers from the Graz University of Technology, the Know-Center GmbH research center, the Johannes Kepler University of Linz, the University of Innsbruck (all from Austria), and the University of Utrecht (Netherlands) wanted to test how accurate the recommendations generated by these algorithms are, especially for listeners of music that is not very popular or not so well known to the general public.
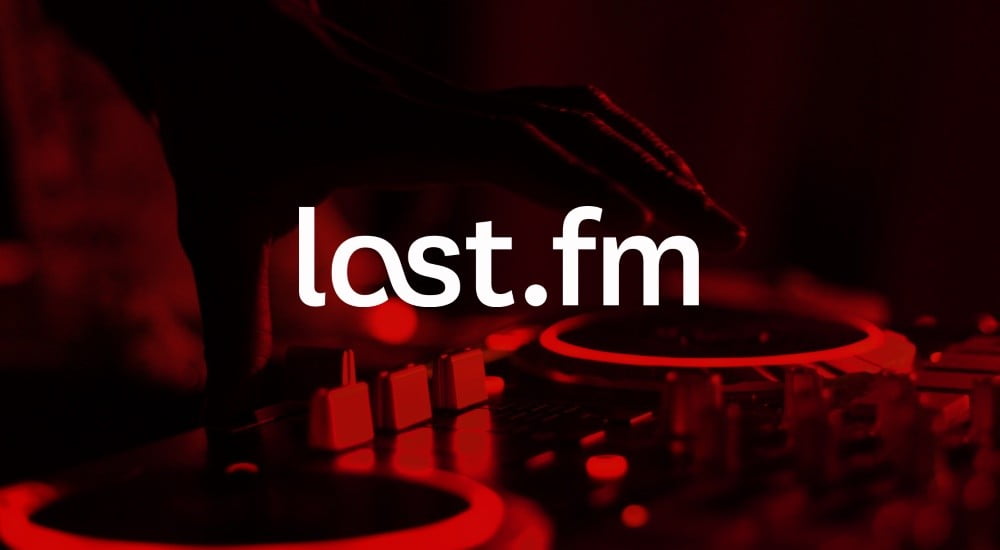
The main result, published in the latest issue of the journal EPJ Data Science, is that these algorithms fail significantly more with hard rock and hip-hop listeners than with other music genres.
A test on Last.fm users revealed the situation
To test this, the team took the listening history of 4148 users of the Last.fm platform, both listeners who tend to listen to more commercially popular music and those who prefer somewhat lesser-known artists (2074 users in each group).
Based on the artists most listened to by each user, the research employed a computational model to predict whether they would like a new song or artist using four different recommendation algorithms. In this way, they confirmed that listeners of popular music tend to receive more accurate and precise recommendations than the less commercial group of listeners.
The authors then categorized listeners of non-commercial music into four groups, according to the characteristics of the music they most often listen to. These groups were: listeners of music genres containing only acoustic instruments, such as folk or singer-songwriters; highly energetic music such as punk or hip-hop; highly acoustic but voiceless music such as ambient music; and highly energetic but voiceless music such as electronica. The research was thus able to compare the histories of each group and identify, with the computational model, which users were more likely to listen to music outside their preferences and the diversity of music genres within each group.
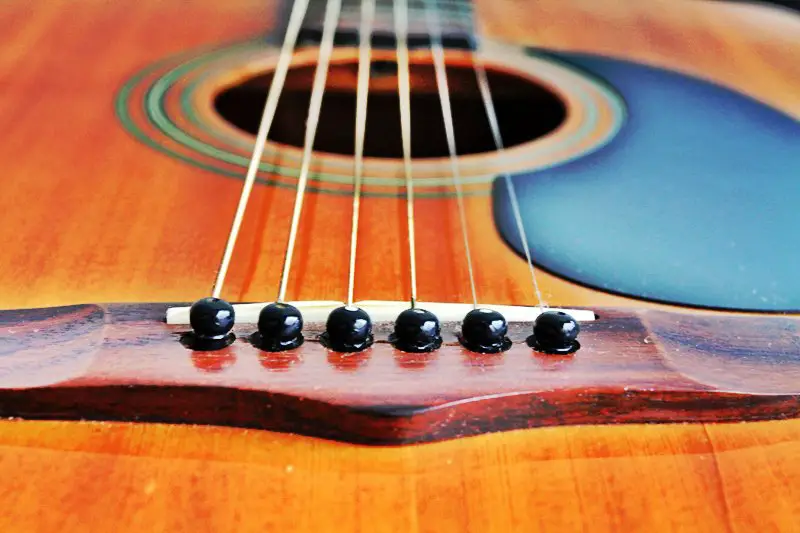
Acoustic music listeners get better recommendations
Through this categorization, the study found that listeners of acoustic music without vocals also tended to prefer songs from the other three groups (energetic, energetic without vocals, and acoustic) and received more accurate recommendations from the computational model. At the same time, the group of energetic music listeners received the worst recommendations from the algorithms, despite the fact that their group featured the greatest variety of music genres-hard rock, punk, hardcore, hip-hop, and pop-rock.
Elisabeth Lex, the co-author of the paper and associate professor of applied computer science at the Graz University of Technology, stresses that music recommendation algorithms are already “essential” for users who want to search, select and filter music app collections.
Despite this, he points out that algorithms can fail to make recommendations for listeners of uncommercial music. “This may be because these systems are biased towards more popular music, resulting in artists outside the mainstream being less listened to,” he notes.
Finally, the authors suggest that their findings could serve as a basis for creating music recommendation systems that provide more accurate recommendations. They caution, however, that their analysis is based on a sample of Last.fm users, which may be unrepresentative for this or other music platforms.