A new player has entered the AI scene and started a new debate, LPU vs GPU. Groq, with its unveiling of Language Processing Units (LPUs), has brought fresh perspectives to the table. As Groq showcases the impressive capabilities of its LPUs, a lively discussion has emerged comparing them to Graphics Processing Units (GPUs), which have long been the go-to for AI tasks.
With Groq’s LPUs setting new standards in processing speed and efficiency, many wonder: Could LPUs revolutionize AI hardware, challenging the dominance of GPUs? To understand this, first, understand what LPU means and explore the differences between LPUs and GPUs.
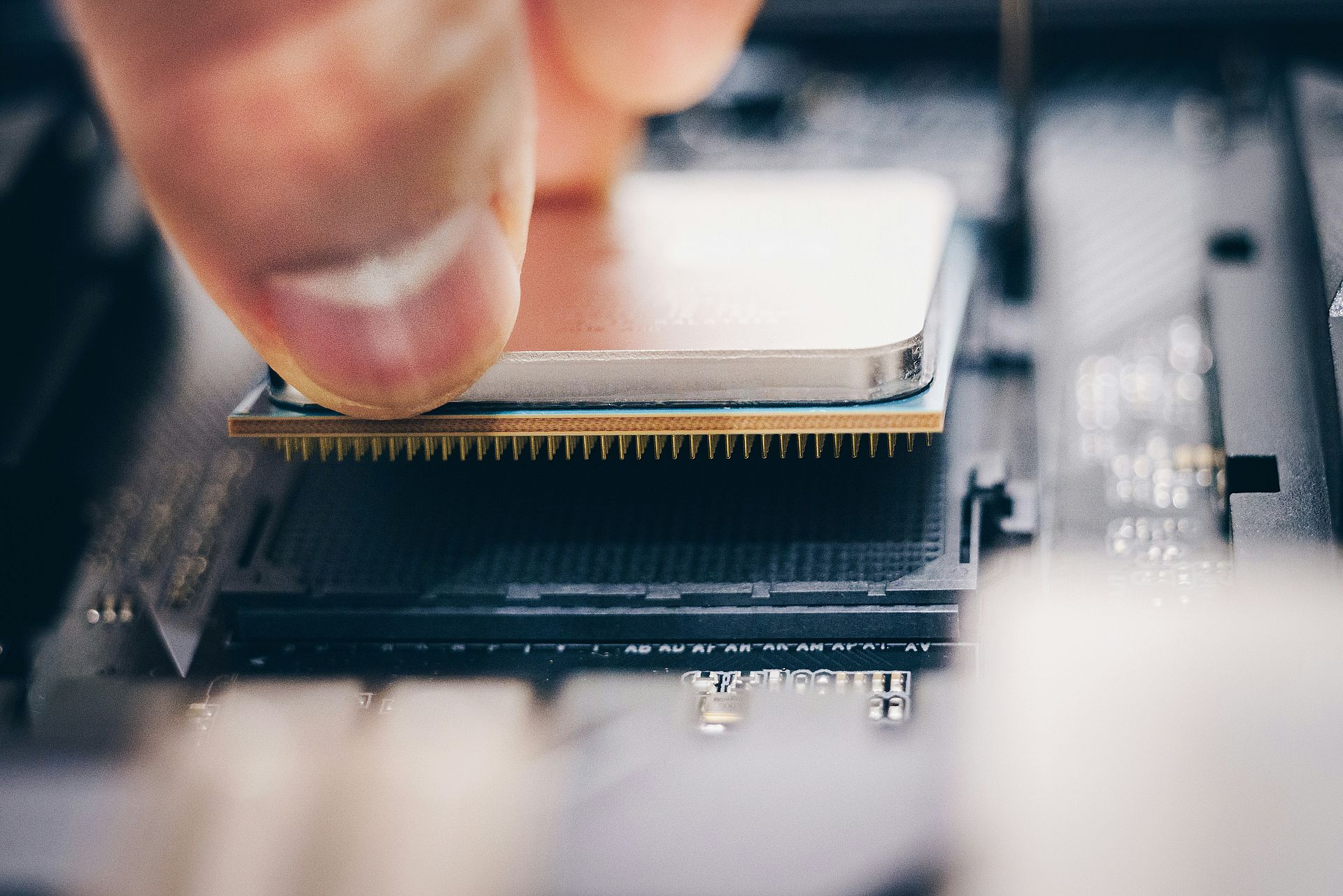
What is LPU?
A Language Processing Unit (LPU) is a specialized hardware component designed specifically for natural language processing (NLP) tasks. LPUs are optimized to efficiently handle operations related to understanding and generating human language, such as text interpretation, sentiment analysis, translation, and content generation.
LPUs differ from traditional processors like CPUs (Central Processing Units) and GPUs (Graphics Processing Units) in that they are specifically tailored to address the unique computational requirements of NLP tasks. They typically feature architectures optimized for sequential processing, which is crucial for accurately processing language-based data.
LPUs have gained attention in the field of artificial intelligence (AI) and machine learning due to the increasing demand for efficient processing of large-scale language models, such as those used in natural language understanding, dialogue systems, and language translation. They offer performance, energy efficiency, and scalability advantages for handling NLP tasks, making them valuable components in AI hardware architectures. But can it take the throne of GPUs?
LPU vs GPU: The comparison you are waiting for
In the world of AI hardware, two key players stand out nowadays: Language Processing Units (LPUs) and Graphics Processing Units (GPUs). LPUs are tailor-made for understanding and generating human language, while GPUs were originally designed for gaming graphics but now handle various tasks. So, what are they good at, what are they not, and where do they shine brightest? Here is what you need to know about LPU vs GPU:
Feature | LPUs (Language Processing Units) | GPUs (Graphics Processing Units) |
---|---|---|
Design Purpose | Specifically designed for natural language processing (NLP) tasks, such as text interpretation and generation. | Originally created for rendering graphics in video games, now used in various applications including AI, machine learning, and graphics rendering. |
Architecture | Optimized for sequential processing, crucial for accurately understanding and generating human language. | Built for parallel processing, allowing execution of multiple tasks simultaneously. |
Advantages | – Specialized for NLP tasks, offering superior performance in language-based operations. – Efficiency in processing language models, potentially reducing time and energy footprint. |
– Versatile across a wide range of tasks including AI, gaming, and graphics rendering.
– High parallel processing capability accelerates complex simulations and deep learning model training. |
Limitations | – Limited application scope beyond NLP tasks due to specialization.
– Emerging technology with challenges in widespread support and availability. |
– High energy consumption, especially during high-performance tasks, leading to challenges in power efficiency.
– General-purpose design may lack efficiency for specific AI tasks compared to specialized processors. |
Suitable for | NLP tasks such as translation, content generation, sentiment analysis, and text summarization. | AI and machine learning tasks, gaming, video rendering, complex simulations, deep learning model training, and more. |
Energy Efficiency | Potentially higher due to optimization for specific tasks, leading to reduced energy consumption in NLP operations. | Lower due to high-performance tasks and parallel processing, resulting in higher energy consumption, especially during intensive computations. |
In summary, LPUs and GPUs have distinct strengths and weaknesses, making them suitable for different types of tasks. LPUs specialize in NLP operations and offer potential energy efficiency advantages, while GPUs are versatile processors capable of handling various tasks but may consume more energy.
So, can LPUs succeed?
LPUs have the potential to change AI hardware by giving GPUs a run for their money. LPUs are great at handling language tasks like understanding and generating human language. They’re really good at tasks like translation and content creation. While GPUs are versatile and can do many things, LPUs might be better for certain jobs because they’re optimized for language tasks. However, GPUs still have the upper hand in doing lots of different tasks and are more widely used. So, LPUs could shake things up in AI hardware, but it’s still early to say for sure.
Featured image credit: Timur Garifov/Unsplash