Microsoft’s take on text-to-speech synthesis, Microsoft VALL-E has been announced in a paper published by the company. The audio model only requires a 3-second audio file to process the given input.
Microsoft VALL-E, a novel language model approach for text-to-speech synthesis (TTS) that leverages audio codec codes as intermediate representations, was just launched by Microsoft. It was pre-trained on 60,000 hours of English speech data and then displayed in-context learning abilities in zero-shot circumstances.
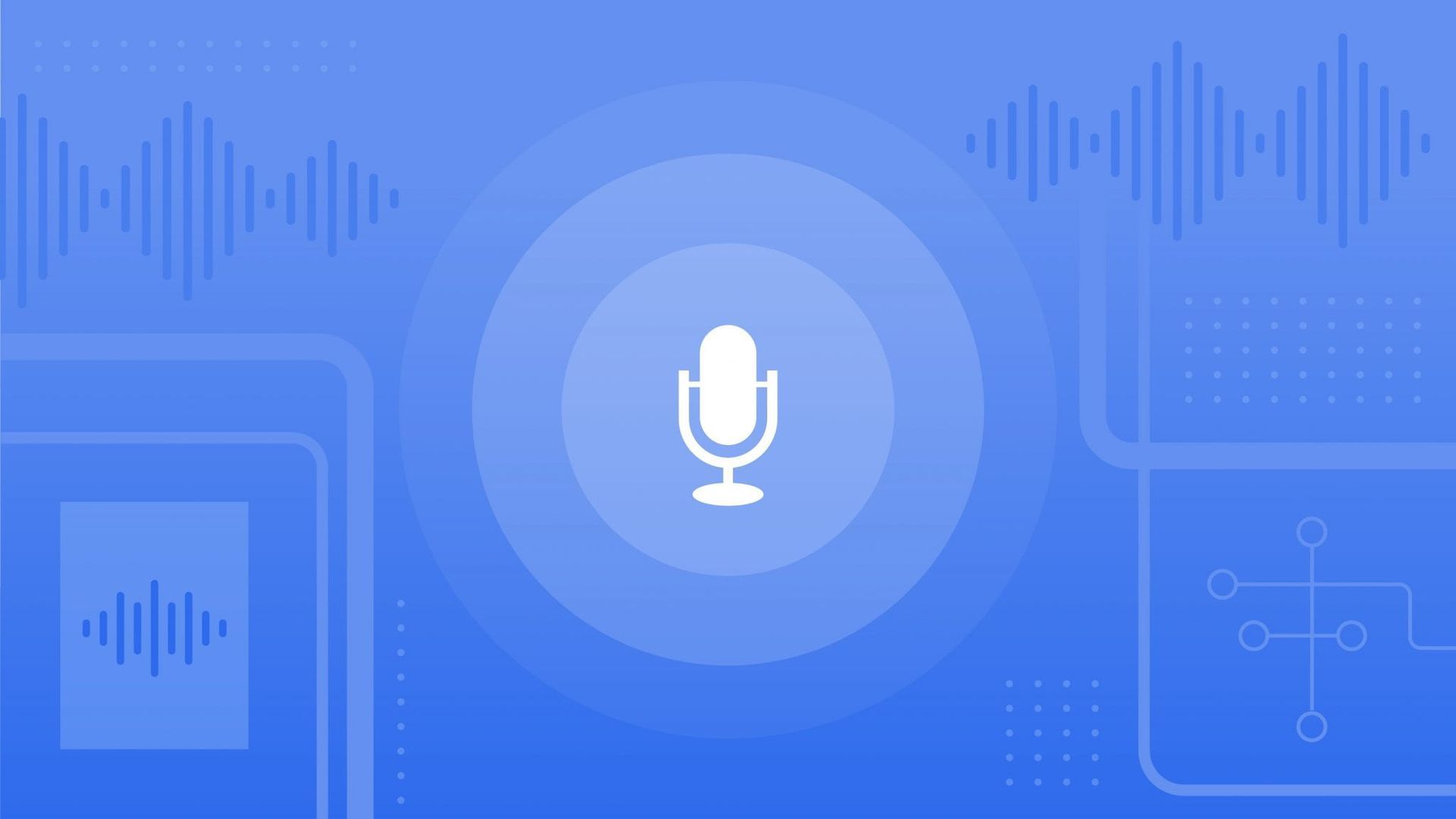
Microsoft VALL-E can produce high-quality personalized speech with just a three-second enrolled recording of an oblique speaker acting as an acoustic stimulus. It does so without the need for additional structural engineering, pre-designed acoustic features, or fine-tuning. It supports contextual learning and prompt-based zero-shot TTS approaches. It appears that the scaling up of semi-supervised data for TTS has been underutilized because Microsoft has used a significant amount of semi-supervised data to construct a generalized TTS system in the speaker dimension.
What can you do with Microsoft VALL-E?
According to the researchers, Microsoft VALL-E is a “neural codec language model” that was trained using discrete codes that were “derived from a pre-existing neural audio codec model.” It was trained on 60 thousand hours of speech, which is “hundreds of times greater than existing systems,” according to the statement. These examples are convincing in contrast to prior attempts that are very obviously robots, even though AI has been around for a while that can realistically mimic human speech.
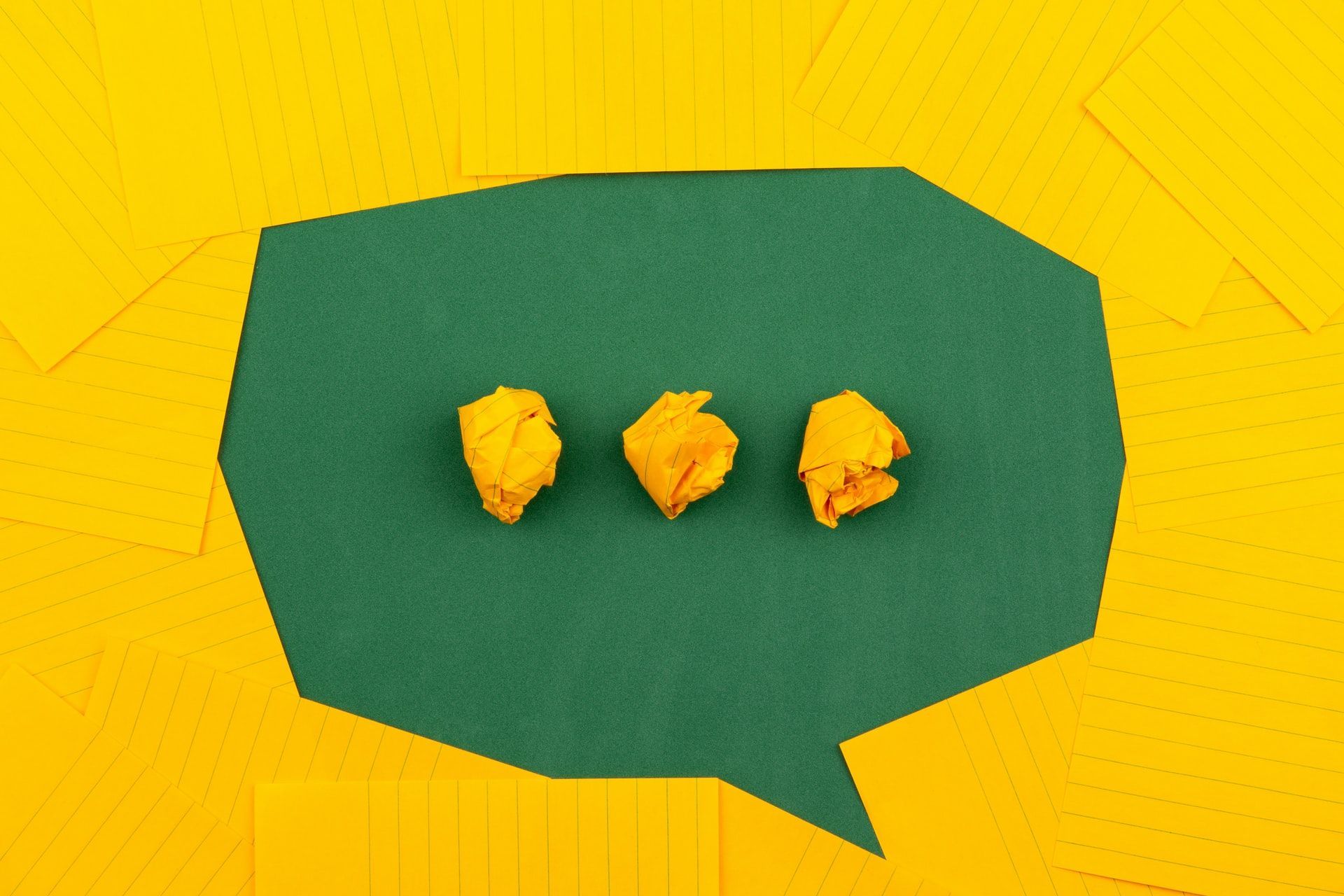
Microsoft VALL-E can “preserve the speaker’s emotion and auditory environment,” according to the researchers, of the prompt. Although it is impressive, technology is still a long way from replacing voice actors because finding the appropriate tone and emotion during a performance is different. Even an advanced version of Microsoft VALL-E wouldn’t be able to perform as well as a skilled professional, yet businesses often prioritize cost-effectiveness over quality.
On Microsoft’s GitHub demo, you can listen to some of the samples.
Microsoft VALL-E features
Although Microsoft VALL-E is very new, it already has many features.
Synthesis of diversity: Because Microsoft VALL-E generates discrete tokens using the sampling-based technique, its output varies for the same input text. It may therefore synthesize different personalized speech samples using a variety of random seeds.
Acoustic environment maintenance: Microsoft VALL-E can provide customized speech while maintaining the acoustic environment of the speaker prompt. In comparison to the baseline, VALL-E is trained on a big dataset with more acoustic variables. The audio and transcriptions were produced using samples from the Fisher dataset.

Speaker’s emotion maintenance: Using the Emotional Voices Database as a resource, for example, audio prompts, Microsoft VALL-E may create customized speech while maintaining the emotional tenor of the speaker prompt. Traditional approaches train a model by correlating the speech to transcription and an emotion label in a supervised emotional TTS dataset. VALL-E can keep the emotion in the prompt even in a zero-shot situation.
Microsoft VALL-E still has issues with model structure, data coverage, and synthesis robustness.
How does Microsoft VALL-E work?
Microsoft used LibriLight, an audio library put together by Meta, to train VALL-voice E’s synthesis skills. Most of the 60,000 hours of English-language speech are taken from LibriVox public domain audiobooks and spoken by more than 7,000 people. The voice in the three-second sample must closely resemble a voice in the training data for VALL-E to get a satisfactory result.

Microsoft offers dozens of audio examples of the AI model in action on the VALL-E example page. The “Speaker Prompt,” one of the samples, is the three seconds of audio that VALL-E is instructed to mimic. The “Ground Truth” is a previously recorded excerpt from that speaker that is used as a benchmark (sort of like the “control” in the experiment). The “VALL-E” sample is the output from the VALL-E model, and the “Baseline” sample is an example of synthesis produced by a traditional text-to-speech synthesis approach.
While Microsoft VALL-E made history as the first, but certainly not the last, major AI project of 2023, the technology giant financially supported OpenAI Point-E, which was published in the last weeks of 2022.