A recent study shows how AI tools can be used for decrypting bacterial virulence networks. Many disease-causing bacteria use a molecular “syringe” to inject a multitude of their proteins, called effectors, into intestinal cells, thus blocking key immune responses.
Now, an international team of scientists from the UK, Israel, and Spain, with the participation of the Polytechnic University of Madrid (UPM), have joined forces to analyze all these protein molecules together, combining laboratory experiments and artificial intelligence (AI) tools.
AI can be used for decrypting bacterial virulence networks
The authors, who publish their study in the journal Science, have used 100 variants of the mouse bacterium Citrobacter rodentium to model the function of the effectors. They found that they work together as a network, allowing the microbe great flexibility to evade the immune system and maintain its pathogenicity.
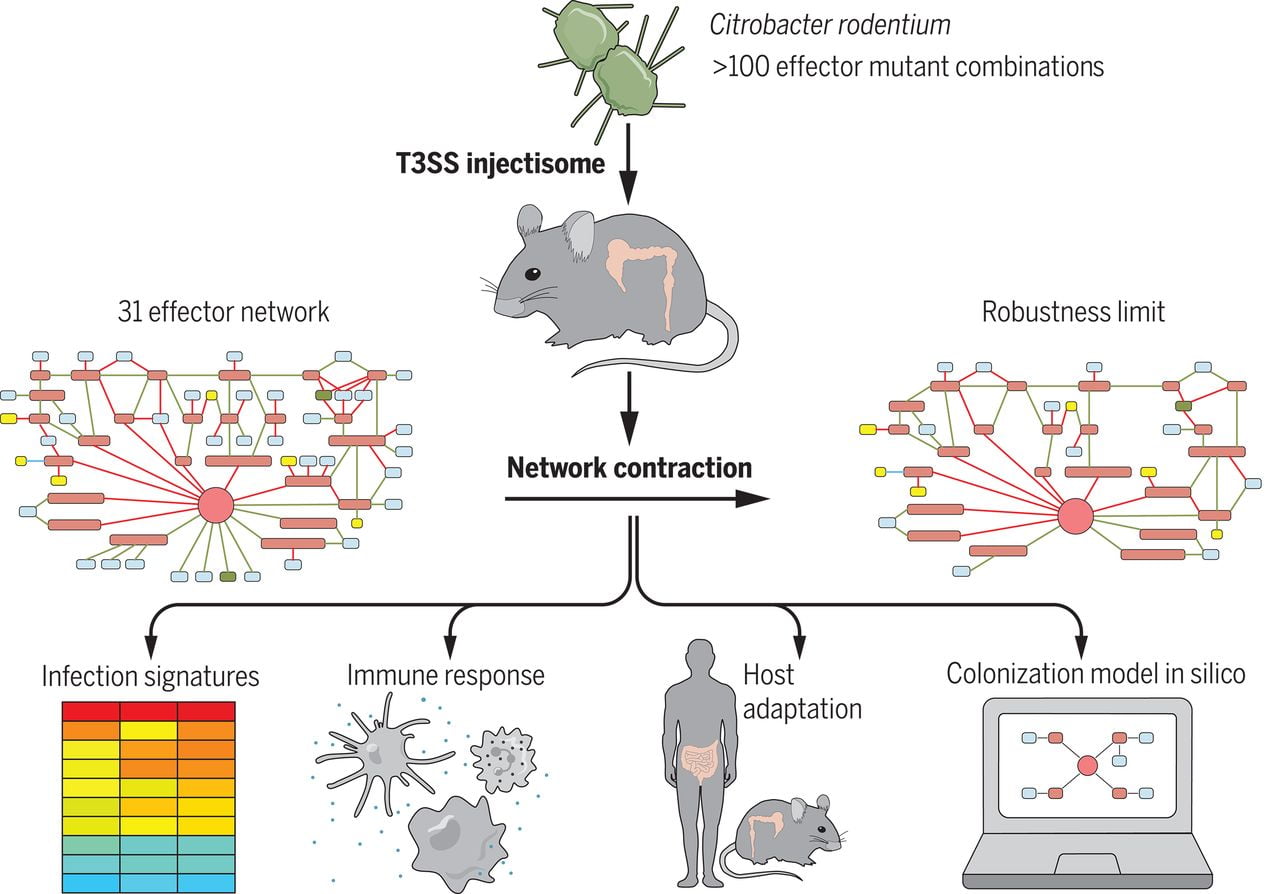
The AI platform correctly predicted the colonization outcomes of alternative networks from the in vivo data. UPM researchers, AI professor Alfonso Rodríguez-Patón and PhD student Elena Núñez Berrueco used the data collected in the lab to build the machine learning model.
The researchers are testing AI tools
The number of possible combinations of effectors exceeds one billion, so studying all the variants would take more than a thousand years of experimental research. That’s where AI comes in to change the rules and make it possible to decipher this complex mechanism. The algorithm developed at the UPM is able to predict the infectious capacity of any variant after learning the patterns of the 100 laboratory experiments.
“By studying such a complex biological system, the AI is able to see what is not obvious to our eyes,” explains Núñez. “The predictions help us identify the most relevant combinations of effectors and thus save time and resources. We can use this model to predict whether and how a new strain, with a different combination of effectors to those studied, can manipulate our cells.”
The algorithm is inspired by artificial neural networks but incorporates knowledge about the targets of the effectors. The architecture of this network has a peculiarity: instead of being generic, it has the same shape as the network of biological interactions of effectors with the components of our cells. This has made it possible to train the network with a very small number of cases, resulting in a model with interpretable results (so-called explainable AI).
With the help of the model, the scientists were able to direct further experiments to the most interesting variants. Thus, they have been able to discover small groups of these molecules that are essential. This means that, when they are eliminated or blocked, the bacteria do not infect, providing a promising target for future treatments to help defeat these nimble invaders.
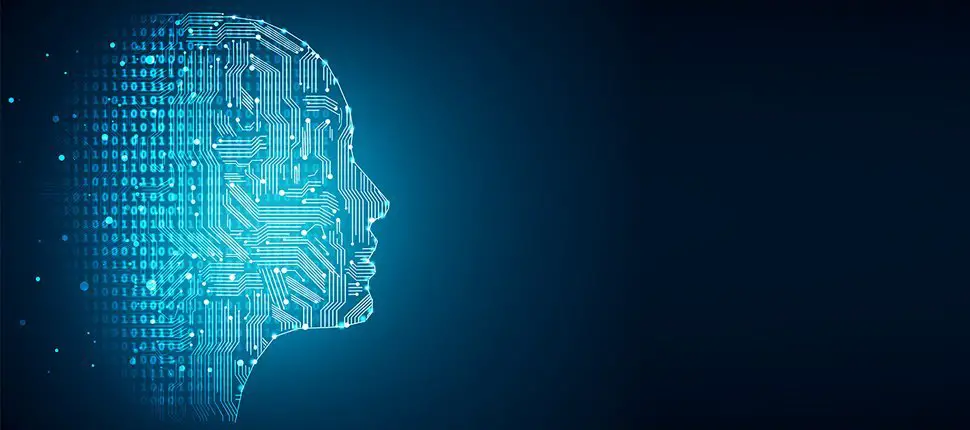
New therapies might be on the way
In fact, the authors also observed that the host mouse is adaptive, being able to circumvent the obstacles erected by the different effector networks and activate complementary immune responses that eliminated the pathogen and induced protective immunity.
Rodríguez-Patón concludes: “Artificial intelligence is once again proving to be a disruptive technology, in this case in the field of microbiology. This interdisciplinary research has required us to develop novel AI techniques to unravel the complex network of molecular signals that bacteria use to infect us. The results obtained are very satisfactory, so we will continue to collaborate with Gad Frankel’s group -one of the lead authors- at Imperial College London in future research.”